Agile-online.org
Huerta, Schade, Granell (Eds): Connecting a Digital Europe through Location and Place. Proceedings of the AGILE'2014 International Conference on Geographic Information Science, Castellón, June, 3-6, 2014. ISBN: 978-90-816960-4-3
Analysing spatiotemporal patterns of antibiotics prescriptions
Luise Hutka and Lars Bernard
Technische Universität Dresden
Professorship of Geoinformation Systems
Dresden, Germany
Abstract
The emergence of antibiotic resistances due to antibiotic residues in urban sewage systems is becoming an increasingly important issue.
This paper presents a model for the spatiotemporal analysis of antibiotic inputs to derive spatiotemporal distribution patterns which are the basis for later predictions of future antibiotic inputs into the sewer system. To identify spatiotemporal distribution patterns of antibiotic prescriptions data statistical and GIS methods like time series and spatial cluster analysis are used. In order to find possible interrelationships the prescription data is combined with other influencing parameters (e.g. cases of respiratory infections) and tested for statistical correlations. Results show a pronounced seasonal course for three antibiotics of the macrolide group which also show high correlations with cases of respiratory infections in the study area. Further, results show that weekly data of respiratory infections by
Google Flu Trends may be used as predictor variable to derive forecasts of future antibiotic inputs into the sewer system.
Keywords: antibiotic prescriptions, spatiotemporal pattern recognition, drug residues, correlation analysis
statistical tools and GIS. The paper starts in describing the study design to analyse spatiotemporal patterns of antibiotics
Drug residues in sewage are an important issue in the context
input into the sewage system using existing medical
of the objectives of wastewater treatment [9, 12, 13]. In
prescription data and further geodata. The remainder focuses
general, it is assumed that an increased input of antibiotics
on discussing the current results and on detecting appropriate
into the environment promotes the formation of antibiotic-
input variables – e.g. from crowd-sourced data – for a model
resistant bacteria. If a pathogen is resistant to a particular
to predict antibiotic release into the sewer system.
antibiotic, taking this antibiotic in case of an infection with the
pathogen is ineffective. In the end, the increasing antibiotic
resistances together with the decreasing development of new
Spatiotemporal Analysis of Antibiotic
antibiotics in recent years may lead to more and more
Medications
antibiotics becoming ineffective, with the result that infectious diseases could spread again [19]. Thus, there are numerous
Various studies demonstrate GIS to be a powerful tool for
studies on the efficiency of various procedures regarding the
analysing spatial and temporal distribution patterns of drug-
specific behaviour of antibiotics and resultant antibiotic
related health data. Cheng et al. [2] used local spatial
resistance [1, 16, 17].
association statistics to examine the geographic variation of
The project ANTI-Resist1 [5] researches the release of
cardiovascular drug-prescribing patterns in Taiwan. Modarai
antibiotics and potentially related appearance of antibiotic
et al. [15] performed a local Moran's I analysis on annual
resistances in the urban sewage system of the city of Dresden.
opioid prescription sales aggregated by 3-digit zip codes and
As a long term objective the project is meant to support the
correlated this data with official data on opioid overdoses. As
design of strategies to reduce the formation of antibiotic
in the previous studies, also in the present work local
resistances in urban wastewater. The project focuses on the
Moran's I analysis is performed on prescription data, but in
development of corresponding monitoring and warning
applying a higher temporal and spatial resolution as the used
systems. Within the project various aspects related to
antibiotic prescription data are available at a weekly/monthly
antibiotic fluxes and transports in urban wastewater systems
resolution for 8 years and on an urban district level. A related
are considered. First, the antibiotic prescriptions of medicines
work on antibiotic prescriptions was published by Kern et al.
are investigated. Second, models are being developed to
[11] who studied the regional variation in outpatient antibiotic
describe their release and transport within the sewage system
use within Germany. In contrast to the work presented here,
and third, the related emergence of antibiotic resistances
Kern et al. analysed prescription data for only one year and on
within the sewer and the water treatment facilities are studied
the large-scale federal state level, further they did not apply
using different measurement and observation methods.
GIS-based spatial analysis.
This paper describes the approach of spatiotemporal
The ANTI-Resist project follows a twofold approach in
analysis and modelling of antibiotic prescriptions using
analysing spatiotemporal patterns of antibiotics emergence and related processes in urban wastewater: (1) Sewer
measuring campaigns and related laboratory studies are
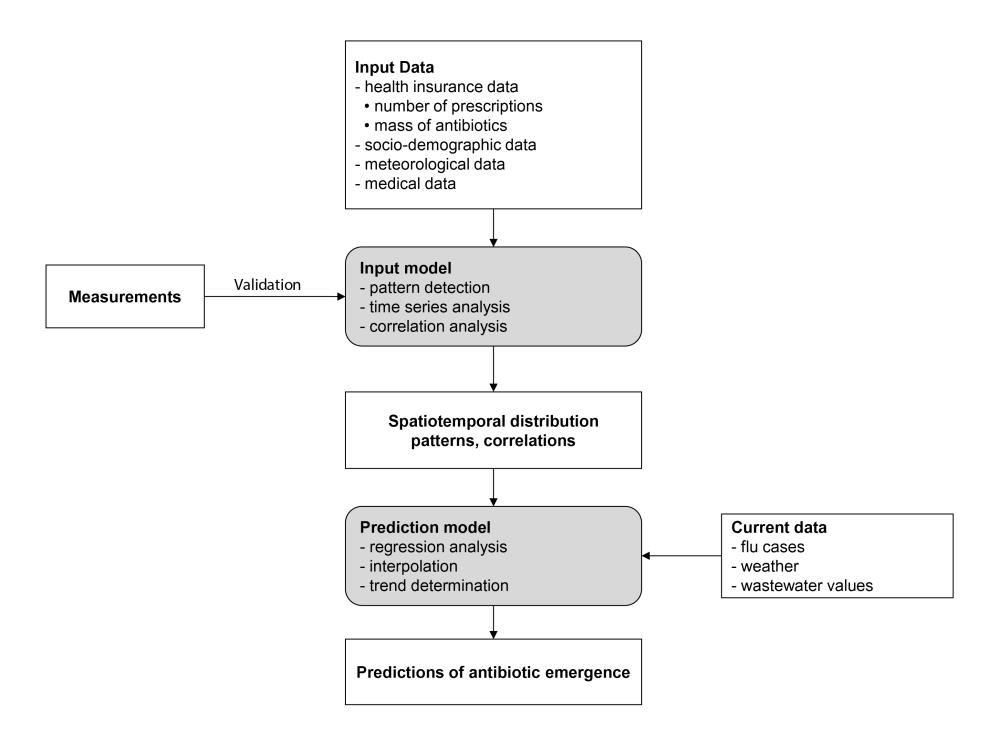
AGILE 2014 – Castellón, June 3-6, 2014
Figure 1: Schema of input model and prediction model
conducted to better understand the actual antibiotic fluxes and
Input Data
the genesis of antibiotic resistance within the sewer system and (2) data driven (statistical) models are getting designed to
Basis for the input model are data about the ambulant
serve as best estimated guesses on where antibiotics are being
antibiotic prescriptions that have been provided by one of the
released and how they are transported through the sewage
German compulsory health insurances (AOK PLUS). These
system. Figure 1 sketches the conceptual frame for these
data are available in a weekly and monthly temporal
models and consists of two components:
resolution for the period from 2005 to 2012. The data are
An input model has been designed to analyse
provided for the 64 urban districts of the city of Dresden and
historical prescription data, socio-economic data
aggregated into three age groups: 0 to 14 years, 15 to 64
and environmental data to identify typical
years, 65 and more years. The following antibiotic substances
of various active ingredient groups are examined: amoxicillin,
medications, to estimate related antibiotic fluxes
azithromycin, cefuroxime, ciprofloxacin, clarithromycin,
into to the sewer system and to evaluate these
levofloxacin-ofloxacin,
estimates against different measurements within the
The input model then serves as the basis for a
Several issues arose when analysing the prescription data
prediction model to estimate the future release and
from the health insurance. First, the supplied data comprise
fluxes of antibiotics into the sewer system. The
only the patients of this single insurance company, which
prediction model shall serve to alert the urban waste
represent about 41% of the population of Dresden. So the
water treatment and environmental agencies about
prescription data is extrapolated to the total population of
the potential occurrence of antibiotic peaks in the
Dresden. Second, due to medical data protection issues
sewage and the released waste water. As the
prescription amounts between 1 and 3 are anonymised. In the
treatment of antibiotics is not part of the operational
calculations this problem is handled by setting all anonymised
waste water treatment these alerts could also trigger
values to the minimum amount of 1. Third, the data can only
related specific measurements in the sewage plant.
be delivered with a delay of at least one year. Consequently
The main objective of the input model is to use spatial
up-to-date official prescription data are not available for the
statistical tools to investigate the variation of antibiotic inputs
studies. This fact is seriously hampering the prediction of
in time and within the urban districts of Dresden as well as
future antibiotics release into the urban sewer system. To
correlate it with other influencing factors that might have an
overcome the latter problem it was necessary to identify data
impact at the variation of the antibiotic prescriptions. This
of other influencing factors that are related to antibiotic
paper focuses the design and results of the input model and
prescriptions and would eventually serve as a proxy for a
will discuss some initial design ideas for the prediction model.
prediction of antibiotic medication. The identified major influence factors are presented in the following.
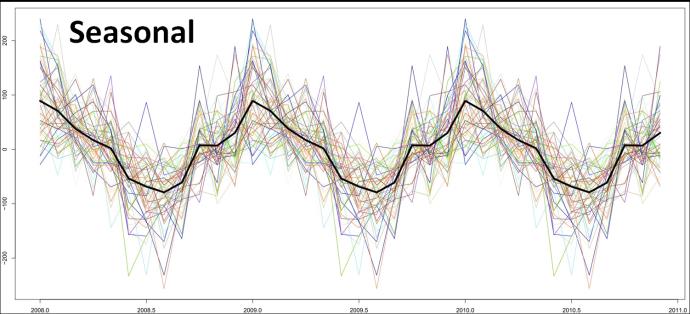
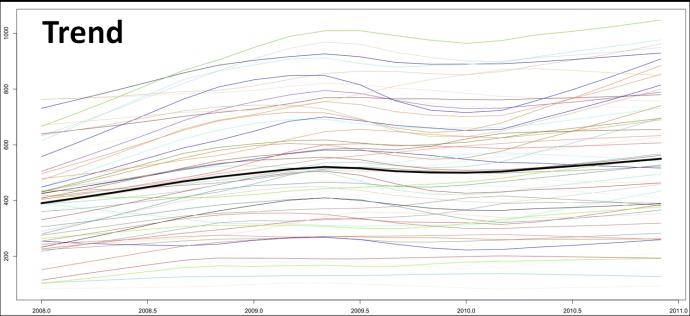
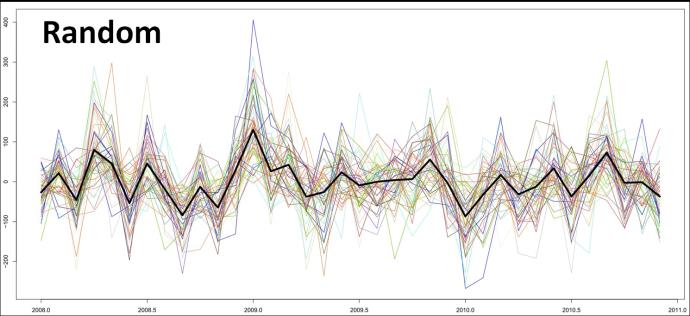
AGILE 2014 – Castellón, June 3-6, 2014
Several studies have shown that there is a correlation
time series into three components: seasonal variation, long-
between respiratory infections and antibiotic prescriptions. On
term trend and random noise (remainder component) [3].
the one hand for the majority of treated cases of respiratory
Thus, the temporal variation of the prescriptions of the
diseases antibiotics are prescribed. This is evident especially
individual antibiotic substances in the overall city as well as in
during the annual cold waves and winter flu season, which
the individual urban districts could be examined as to whether
regularly are accompanied by a significant increase of
there are certain long-term trends and periodic seasonal
antibiotic prescriptions [4, 8, 20]. On the other hand often a
patterns. An example of the result of such a time series
secondary bacterial infection follows a flu infection, as the
analysis is shown in Figure 2.
organism is already weakened due to the fight against the
viruses. Therefore bacteria can more easily lead to further
Figure 2: An example for the resulting components of a time
infections that are then often treated by the use of antibiotics –
series analysis in R using the STL function (sum of
all antibiotic substances, 2008 – 2010)
prophylactically, without bacterial caused symptoms being already present [10, 18]. For these reasons contemporary available representative data about current cases of respiratory diseases have been considered as potential proxies for the input model. Such data is provided by the flu trends portal from Google2. Ginsberg et al. [6] have analysed billions of Google search requests and determined that there is a high correlation between the frequency of particular search terms and the actual number of patients with influenza-like symptoms at a time. For the validation of their results they
used historical data of traditional influenza surveillance systems. For Germany Google Flu Trends provides the weekly cases of respiratory infections per 100,000 inhabitants at a Federal State level from 2003 up to the current week [7].
Other influencing factors that have been identified and
incorporated into the input model are meteorological (temperature, precipitation, etc.) and sociodemographic (population, employment structure) parameters.
For the meteorological parameters the Regional Climate
Information System for Saxony, Saxony-Anhalt and Thuringia
(ReKIS)3 is used. This database contains – for the city of Dresden – data for 3 climate stations and 3 precipitation stations in a daily resolution for the years 1961 to 2013. At the most comprehensive station about 16 parameters are determined.
For the sociodemographic parameters data from the Dresden
statistics office is used4. It provides yearly data at an urban district level about the population, discriminated by sex and nine age groups and data on employees and unemployed persons as relative proportions of the relevant age group. The
health insurance company AOK PLUS provided data on the
age structure of their patients in 5 age groups at the urban
ArcGIS (ESRI ArcGIS 10.2) with its geostatistical methods
district level for the year 2011. Most of the input data
for pattern recognition has been used to analyse the spatial
described here can be explored via the ANTI-Resist
distribution of the antibiotics prescriptions within the Dresden
urban districts. ArcGIS offers several functions for the
analysis of local spatial patterns: Hot Spot Analysis (Getis-
Ord Gi*)6 and Cluster and Outlier Analysis (Anselin Local
Morans I)7. Figure 3 and 4 show examples of the results of the respective cluster method. The Hot Spot Analysis results in
In a first step the antibiotic prescriptions have been modelled
statistically significant clusters of similarly high values
regarding their temporal and spatial distribution using various
(Figure 3 - red) or low values (Figure 3 - blue) for different
statistical and GIS-based methods.
confidence levels. In contrast, the result of the Cluster and
The temporal analysis has been carried out by time series
Outlier Analysis shows not only statistically significant
analysis in R using the STL function, which decomposes a
clusters of high values (Figure 4 - red) or low values
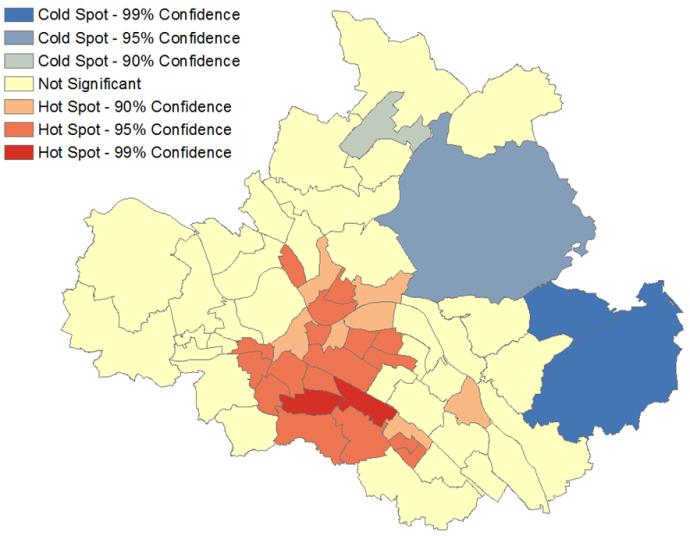
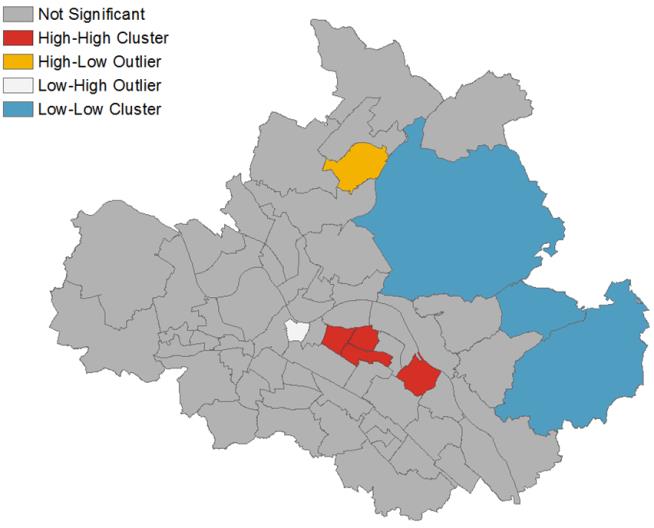
AGILE 2014 – Castellón, June 3-6, 2014
(Figure 4 - blue) but also districts of high values surrounded
by low values (Figure 4 - yellow) and vice versa (Figure 4 - white). As the Cluster and Outlier Analysis seemed more
Time series analysis
meaningful and as it also provides hints to outlier districts, this method has been selected.
The individual antibiotic substances are prescribed differently
In this way, the spatial and temporal prescribing patterns of
throughout the year, depending on the application or bacteria
antibiotics in Dresden and their changes over time have been
causing certain infections. The selection of a suitable
determined. In a next step possible explanations for the
antibiotic is at the discretion of the attending physician. This
occurrence of specific distribution patterns should be
is also reflected in the temporal analysis of the antibiotic
identified. Therefore the antibiotic prescription data are
prescriptions data for the years 2005 to 2011, which showed
analysed to search for statistical correlations (next section)
that the temporal prescription behaviour, especially the
considering the above mentioned influencing factors. The
seasonal course, differs according to the individual antibiotic
correlation analyses are mostly performed via simple linear
substances. While some substances show a more or less
Pearson correlation (using SPSS Statistics 21).
pronounced seasonal pattern (amoxicillin, azithromycin,
The input model still needs to be validated with actual
clarithromycin, doxycycline, roxithromycin), others are
measurements in the sewer system made within the project.
prescribed relatively evenly over the year (ciprofloxacin,
However, a successful validation study strongly depends on
clindamycin) or have no specific pattern (cefuroxime,
the identification of appropriate measuring points that can be
levofloxacin-ofloxacin,
defined as being comparable with the prescription data at the
sulfamethoxazole-trimethoprim). The clearest seasonal pattern
urban district level.
is shown by the antibiotics of the macrolide group
(azithromycin, clarithromycin, roxithromycin) with maximum
Figure 3: Hot Spot Analysis of azithromycin prescriptions in
prescriptions in the winter season and minimum values during
the summer months.
Regarding the trend component, most antibiotics indicate a
decreasing trend. Only amoxicillin, cefuroxime and levofloxacin-ofloxacin reveal an increasing trend, while for azithromycin and clindamycin there is almost no trend apparent.
Cluster and Outlier analysis
The result of the Cluster and Outlier analysis in ArcGIS depends on the selected distance and spatial relationship of the neighbouring features by which the algorithm calculates the clusters. Therefore, the analysis was first carried out with different parameter settings to find the appropriate preferences.
conceptualization
ZONE_OF_INDIFFERENCE is chosen, where a threshold
value specifies whether to include or exclude neighbours and
Figure 4: Cluster and Outlier Analysis of azithromycin
where neighbours are weighted by the Inverse Distance
prescriptions in January 2009
conceptualization of spatial relationship seemed most suitable for the analysis of the antibiotic prescriptions within the Dresden urban districts, as the polygons of the urban districts have different sizes and the (daily) mobility of citizens may lead to movements across several district boundaries. To cope with these cases it is recommended to use a distance-based conceptualization with a smooth transition as by ZONE_OF_ INDIFFERENCE.
The ArcGIS tool Incremental Spatial Autocorrelation8 is
used for choosing an appropriate threshold distance. The tool calculates the spatial autocorrelation for various distances, to determine the distance at which the spatial processes (the clustering) are most pronounced. This resulted in proper threshold distances of 2800 and 6400 meters for the data at hand, depending on the scale considered for the resulting clusters.
AGILE 2014 – Castellón, June 3-6, 2014
Figure 5: Weekly cases of respiratory infections and macrolide prescriptions 2009 – 2011
Source: respiratory infections data: Google Flu Trend; prescription data: AOK PLUS (AOK Sachsen und Thüringen)
The outcome of these analyses provided resulting cluster
Table 1: Pearson correlation coefficients (R) for monthly
maps, which are very different according to the considered
Google Flu Trends data and substance-specific
antibiotic substance and the given point in time. There were
antibiotic prescriptions from 2005 to 2011 (n = 84)
hardly any general patterns for all data. Therefore, the
active ingredient
individual cases have to be considered. Nonetheless, there are
some urban districts, mostly in the central region of Dresden,
that are more often part of a significant cluster than others (see
also Figure 4). Thus, from a sewage treatment perspective,
these cluster areas are the neighbourhoods that should be
given priority and be investigated in more detail.
Levofloxacin-ofloxacin
Correlation analysis
As stated above data about respiratory infections are
Phenoxymethyl penicillin
considered as a proxy for antibiotic prescriptions. A
correlation analysis on the monthly Google Flu Trends data
and the prescriptions for all individual antibiotic substances
has been performed (Table 1). As result there are three
* The correlation is significant at the 0.05 level.
substances presenting a significant strong correlation with the
** The correlation is significant at the 0.01 level.
cases of respiratory infections: azithromycin (r = 0.94,
p < 0.01), roxithromycin (r = 0.83, p < 0.01) and
clarithromycin (r = 0.76, p < 0.01), all belonging to the
Conclusion and Outlook
macrolide group. These three antibiotics show a seasonal pattern similar to the annual wave of influenza.
The presented study succeeded in identifying spatial and
Consequently the correlation analysis for the three
temporal patterns of antibiotic descriptions, offering a
macrolide substances has been repeated with higher resoluted
promising path for future predictions of antibiotic releases in
weekly data to include a wider sample and to create a basis for
urban waste water. This work also demonstrated a statistical
prospective weekly predictions (Figure 5). That way the
significant correlation between respiratory infections and
correlation coefficient has been improved for roxithromycin (r
prescriptions of antibiotic substances of the macrolide group.
= 0.88, p < 0.01) and clarithromycin (r = 0.90, p < 0.01), for
However, it should be noted that it is not known when exactly
azithromycin (r = 0.91, p < 0.01) it remains almost as high as
a respiratory infection occurs within a week, how long it lasts
with the monthly data.
and when exactly any antibiotics are prescribed or a secondary
infection occurs. Moreover, as a recent study pointed to some issues in the usage of Google Flu Trends [14], further
AGILE 2014 – Castellón, June 3-6, 2014
investigation are required to validate the first results,
epidemics using search engine query data. Nature,
presented in this paper. Additional correlation analyses
457(7232): 1012-1014, 2009.
considering other influencing factors as meteorological and
sociodemographic parameters are ongoing.
[7] Google, editor. Google Flu Trends. Frequently asked
Future work will focus (1) on the validation of the input
model using the results of the ANTI-Resist measurement
campaigns and (2) on the development of a prediction model
last accessed 02/2014.
to derive forecasts of the expected antibiotics input into the
sewer system. Predictions will be deduced by the combination
[8] H. Goossens, M. Ferech, R. Vander Stichele and M.
of the findings of the input model with up-to-date information
Elseviers. Outpatient antibiotic use in Europe and
using regression and interpolation functions. Based on the
association with resistance: a cross-national database
shown high correlation between respiratory infections and
study. The Lancet, 365(9459): 579-587, 2005.
macrolide prescriptions, the weekly available Google Flu
Trends data can be integrated into a simple linear regression
[9] T. Heberer. Tracking persistent pharmaceutical residues
model to derive prediction intervals of expected antibiotic
from municipal sewage to drinking water. Journal of
inputs into the sewer system for the same week. Thus, the
Hydrology, 266(3): 175-189, 2002.
final results could be useful for the sewage treatment plant,
which could initiate prompt provisions to increasing
[10] S. Herold. Pathogenese, Klinik und Therapie der
antibiotics input events, as well as for the public health sector,
which could regulate the prescription behaviour of antibiotics
Intensivstation. Pharmazie in unserer Zeit, 40(2): 115-
in the appropriate way.
119, 2011. (In english: Pathogenesis, clinic and therapy
[11] W.V. Kern, K. Nink, M. Steib-Bauert and H. Schröder.
Regional variation in outpatient antibiotic prescribing in Germany. Infection, 34(5): 269-273, 2006.
The project is funded by the Federal Ministry for Education
and Research (BMBF) and is part of the program "Research
[12] K. Kümmerer. Antibiotics in the aquatic environment – a
for Sustainable Development". The fruitful cooperation with
review – part I. Chemosphere, 75(4): 417-434, 2009.
our ANTI-Resist project partners is gratefully acknowledged.
Special thanks go to Daniel Kadner for developing the ANTI-
[13] K. Kümmerer. Antibiotics in the aquatic environment – a
Resist Geoportal.
review – part II. Chemosphere, 75(4): 435-441, 2009.
References
[14] D. Lazer, R. Kennedy, G. King and A. Vespignani. The
Parable of Google Flu: Traps in Big Data Analysis.
[1] A. L. Batt, S. Kim and D. S. Aga. Comparison of the
Science, 343 (6176): 1203-1205, 2014.
occurrence of antibiotics in four full-scale wastewater
treatment plants with varying designs and operations.
[15] F. Modarai, K. Mack, P. Hicks, S. Benoit, S. Park, C.
Chemosphere, 68(3): 428-435, 2007.
Jones, S. Proescholdbell, A. Ising and L. Paulozzi.
Relationship of opioid prescription sales and overdoses,
[2] C. L. Cheng, Y. C. Chen, T. M. Liu and Y. H. K. Yang.
North Carolina. Drug and alcohol dependence, 132(1):
Using spatial analysis to demonstrate the heterogeneity
of the cardiovascular drug-prescribing pattern in Taiwan.
BMC public health, 11(1): 380, 2011.
[16] B. Pauwels and W. Verstraete. The treatment of hospital
wastewater: an appraisal. J Water Health, 4: 405-416,
[3] R. B. Cleveland, W. S. Cleveland, J. E. McRae and I.
Terpenning. STL: A seasonal-trend decomposition
procedure based on loess. Journal of Official Statistics,
[17] G. Qiu, Y. Song, P. Zeng, L. Duan, and S. Xiao.
6(1): 3-73, 1990.
Combination of upflow anaerobic sludge blanket
(UASB) and membrane bioreactor (MBR) for berberine
[4] P. Davey, C. Pagliari and A. Hayes. The patient's role in
reduction from wastewater and the effects of berberine
the spread and control of bacterial resistance to
on bacterial community dynamics. Journal of hazardous
antibiotics. Clinical Microbiology and Infection, 8(s2),
materials, 246: 34-43, 2013.
[18] T. Schaber. Diagnostik, Therapie und Prävention der
[5] Forschungsverbund Public Health Sachsen und Sachsen-
Influenza (Virusgrippe). Pneumologie, 57(01): 27-33,
Anhalt. ANTI-Resist - Online available:
2003. (In english: Diagnosis, Therapy and Prevention of
last accessed 02/2014.
[6] J. Ginsberg, M. H. Mohebbi, R. S. Patel, L. Brammer,
[19] B. Spellberg, R. Guidos, D. Gilbert, J. Bradley, H. W.
M.S. Smolinskiand L. Brilliant. Detecting influenza
Boucher, W. M. Scheld, J. G. Barlett and J. Edwards.
AGILE 2014 – Castellón, June 3-6, 2014
The epidemic of antibiotic-resistant infections: a call to action for the medical community from the Infectious Diseases Society of America. Clinical Infectious Diseases, 46(2): 155-164, 2008.
[20] S. Stone, R. Gonzales, J. Maselli and S. R. Lowenstein.
Antibiotic prescribing for patients with colds, upper respiratory tract infections, and bronchitis: a national study of hospital-based emergency departments. Annals of emergency medicine, 36(4): 320-327, 2000.
Source: https://agile-online.org/Conference_Paper/cds/agile_2014/agile2014_111.pdf
Kein Pressedienst - Nur zur Information Zusammengefasste Meldungen aus: Daily/Sunday News (DN), The Guardian, Sunday Observer, ITV Habari, Nipashe, The Citizen, ThisDay, Arusha Times, Msema Kweli, The East African, Uhuru na Amani (Zeitschrift der ELCT), UN Integrated Regional Information Networks (IRIN) und anderen Zeitungen und Internet Nachrichtendiensten in unregelmäßiger Auswahl
HERZIENING RICHTLIJN HOGE BLOEDDRUK KWALITEITSINSTITUUT VOOR DE GEZONDHEIDSZORG CBO In samenwerking met: • Institute for Medical Technology Assessment• Nederlandsche Internisten Vereeniging• Nederlandse Hartstichting• Nederlandse Vereniging voor Cardiologie• Nederlands Huisartsen Genootschap• Nederlands Hypertensie Genootschap• Vereniging voor Epidemiologie